Understanding the first component of Machine Learning Models

To enable your business processes with Machine Learning, you must consider both the data and the model. We’ve already discussed data extensively — in my last article on “data collection and feature extraction for Machine Learning”. That means, so far, we covered:
- Basic concepts and terminology related to data collection;
- Preparing and collecting data;
- Identifying and extracting features.
In this article, I will discuss the Machine Learning Model, which involves two components: ML Algorithm and ML Training. After a quick glance at their definitions, I’ll focus on Machine Learning Algorithms. Training will be the topic of my next post.
Algorithms and Types of Machine Learning
There are several ways to approach Machine Learning Models. In this section, I’ll talk about how the algorithms correspond to the different types of Machine Learning; i.e., Supervised, Unsupervised, and Reinforcement Learning.
Supervised Learning
Supervised Learning means that the machine learns from a set of “labeled” or “tagged” training data with corresponding outputs. We can think of this in a simpler way, using f(x) = y. In this function, f is the machine learning model, x is the input data, and y is the output data.
There are three common methods of Supervised Learning: Binary Classification, Multiclass Classification, and Regression. Let’s discuss and look at some examples of each one.
1. Binary Classification
Binary Classification, a basic and common method, simply uses two categories: “Positive or Negative”, “0 or 1”, “Yes or No”, “Good or Bad”.
It can be applied broadly to many real-life scenarios. For example, when an email comes in, a binary classification system will analyze the email’s content and categorize it into “Spam” or “Non-spam”.
Some common binary algorithms are:

2. Multiclass Classification
Multiclass Classification sorts the input data into different categories, making it quite similar to Binary Classification. However, as the name implies, Multiclass Classification provides more than two categories.
Let’s say we train the system to identify fruits, such as oranges, apples, bananas, and lemons. Then, when you randomly input a fruit photo to the system, it will tell you the correct fruit name.
Some common multiclass algorithms are:
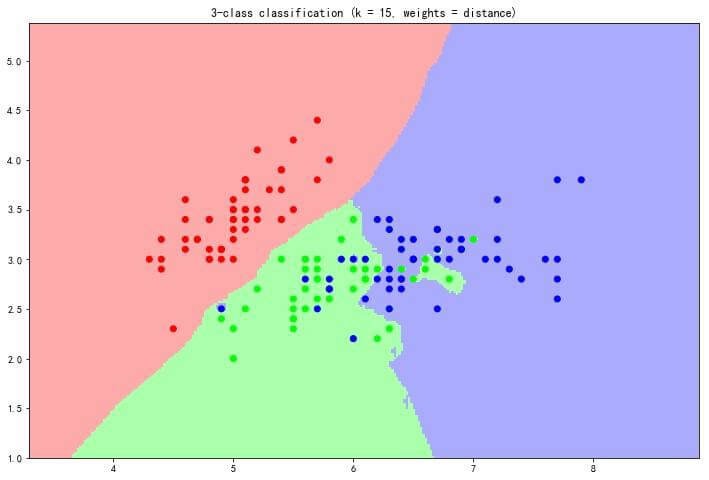
3. Regression
Regression predicts a continuous value based on the input data. The difference between Regression and Multiclass Classification is that Regression returns a “value” or “number” where Multiclass Classification returns a “category” or “class”.
As an example, a regression can be used in the financial sector. If you provide historical stock prices to train an algorithm, it can be used to predict future stock prices on any given date.
Some common algorithms are:
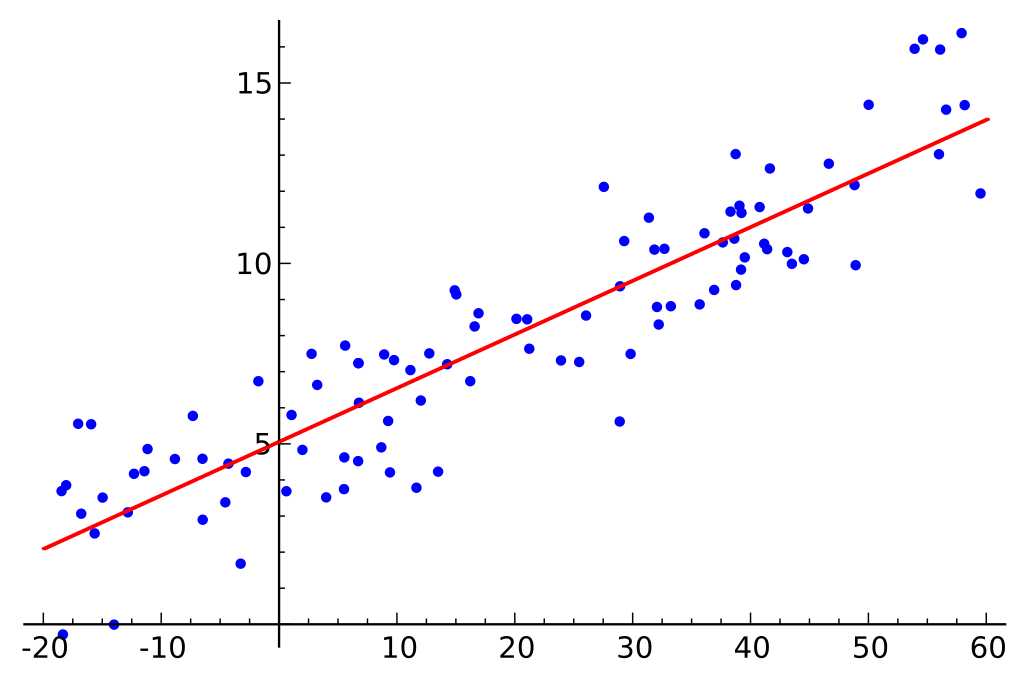
Unsupervised Learning
Unsupervised Learning is the opposite of Supervised Learning. Even if you don’t provide a set of “labeled” or “tagged” training data, the machine will still help you to discover the unknown patterns within.

Clustering algorithms are used in Unsupervised Learning to classify data into different groups.
Several common clustering algorithms are:
- K-means,
- Hierarchical,
- Mean-Shift,
- Density-Based Spatial Clustering of Applications with Noise (DBSCAN).
Many e-commerce companies use Unsupervised Learning for item recommendation. For example, an e-commerce company might use Unsupervised Learning to classify a user segment, and, according to the segment, the system will push specific items to them to increase the purchase conversion rate.
Reinforcement Learning
Reinforcement Learning, often referred to simply as RL, is a relatively new method of Machine Learning.
It started in 1997, when IBM’s supercomputer, Deep Blue, beat Garry Kasparov in chess. It was the first computer ever to beat a world champion and a stunning debut for Reinforcement Learning — which is how Deep Blue learned chess.
RL differs from other types of learning in that it provides an initial state. The ML agent can then select from a number of actions and move to different states, receiving positive or negative rewards for each. Through this process, it teaches itself the best policy to maximize rewards over time.
It can be applied to self-driving cars in the automotive industry.
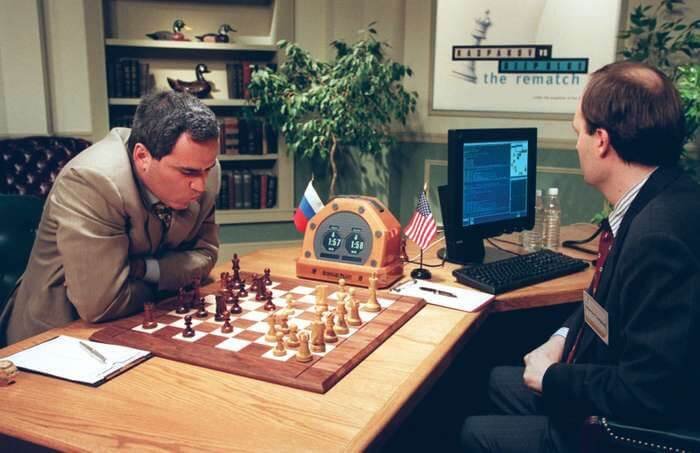
There are two common approaches to Reinforcement Learning: Model-Based and Model-Free.
The Model-Based approach uses planning to decide what action to take; conversely, the Model-Free doesn’t like planning ahead an instead uses “trial-and-error”.
RL is a fairly new area of Machine Learning and too complicated to cover in detail here. However, a colleague of mine is publishing an extensive series on Reinforcement Learning, which you can start following here.
In Conclusion
You’ve learned some basic information about the Machine Learning Algorithms used in Supervised, Unsupervised and Reinforcement Learning.
You should also have an idea about how they can be applied in practical situations.
In my next article, I will elaborate on ML Training to round off our discussion of Machine Learning Models.
Thank you for reading! Follow me here and on social media to make sure you don’t miss the next installment. If you found this article useful, a share and some claps would mean the world to me and help fuel the rest of my series.
Questions or comments? I’d be more than happy to answer them here or via email.
You can also find me on LinkedIn, Facebook, Instagram, and my personal website.