How to uncover relevant patterns in large amounts of data

If we want machines to act and think like humans, we need to look at how we learned to walk and talk in the first place.
The answer, of course, is data. Babies learn by absorbing a whole lot of information (data) and analyzing it to identify similarities and patterns.

In my previous article on identifying business processes that can be ML-enabled, I showed how to find out which of your processes can benefit from machine learning.
The next step to enabling Machine Learning is data — because, without data, there is no machine learning.
When you think about data, many questions emerge:
- Where is your data?
- How much data do you need?
- What kind of data should you collect?
- What are the data patterns (features)?
- How can you identify and extract features from data for Machine Learning?
Let’s address each of these questions so you can apply them to your business one by one.
Preparing and Collecting Data
Where is your data?
Data may be stored in your application database or by other third-party service providers.
If you want to, for example, analyze your users’ spending behavior, you may need to pull out their purchasing records from your own database.
Conversely, if you want to understand user interests, you might need to find third-party service providers who specialize in generating such content.
How much data do you need?
This is an interesting question, but it has no definite answer because “how much” data you need depends on how many features there are in the data set (which we’ll cover in the next section).
I do recommend collecting as much data as possible. Feature selection will help you to filter out the useful nuggets from your big data. Regardless, “big data” will take more time to analyze.
What kind of data should you collect?
Data can be categorized into two types: Structured and Unstructured. Structured Data refers to well-defined types of data that are stored in search-friendly databases, while Unstructured Data is “everything” you can collect — but it’s not search-friendly.
Structured Data:
- Numbers, dates, strings, etc.
- Less storage
Unstructured Data:
- Text files and emails
- Media files (videos, music, photos)
- Other large files
According to Gartner, over 80% of an enterprise’s data will be unstructured.
Identifying and Extracting Features
To assist our discussion of data extraction, let’s put down some simple terms.
- Data: all of the information you can collect, which can be Structured or Unstructured
- Data set: your collection of data
- Feature: patterns found in your data set; used to help you extract relevant data for training models
- Model: your Machine Learning algorithm
What are Features?
As defined above, features are the patterns in your data set that can be used to train models. Good features (which we’ll learn to identify in a moment) can help you to increase the accuracy of your Machine Learning model when predicting or making decisions.
Your data set will have many features, but not all are relevant. With feature selection,you can avoid wasting time calculating and collecting useless patterns that you’ll have to remove later.
Feature selection helps you simplify your ML models and enables faster, more effective training by:
- Removing unused data;
- Avoiding “Garbage In Garbage Out”;
- Reducing overfitting;
- Improving the accuracy of a Machine Learning model;
- Avoiding the curse of dimensionality.
Next, let’s talk about methods for feature selection.
How can you identify and extract features from data for Machine Learning?
Now that we’ve covered data collection, it’s time to apply different feature selection methods. This will help you filter useful content from your data for your Machine Learning models.
The three general methods for this are Filter, Wrapper, and Embedded.
Filter Methods

The Filter Method uses statistical calculations to compute scores (or ratings) for all features independent from any Machine Learning model. Based on the scores, you can decide which features you want to keep and which to remove. However, this method ignores the relationship between features themselves.
Wrapper Methods
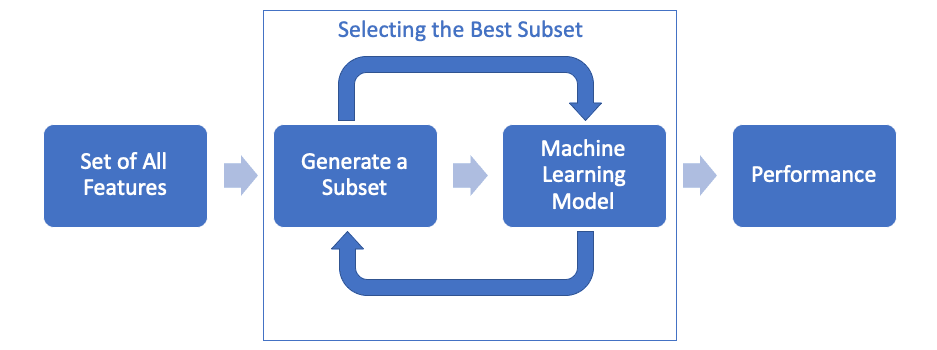
The Wrapper Method will repeat adding/removing features in the subset and use a model to measure its performance until you choose the best one. However, this will cost a lot of time in computation.
Unlike the Filter Method, in which you must use statistical calculations to develop a subset of features, the Wrapper Method uses a real model to pick the best subset of features based on real performance.
Embedded Methods
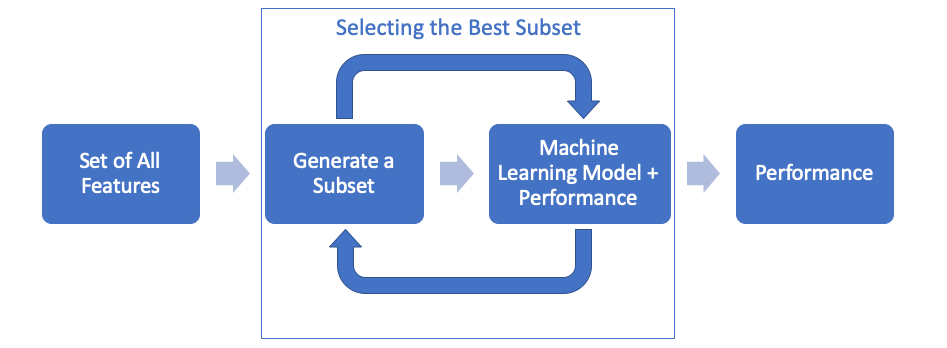
The Embedded Method includes its own feature selection methods such as LASSO Regression or RIDGE Regression. In other words, you don’t need to select features yourself.
In Conclusion
Congratulations, you’ve made it to the end of another article! I hope your understanding of Machine Learning is expanding.
Now that we’ve covered the basics of collecting data and selecting features, let’s recap the ground you’ve gained in my series so far:
- You have a clear definition of Artificial Intelligence and Machine Learning;
- You know how to identify which business processes in your business can be Machine Learning-enabled ;
- You know how to find your data and features from big data for Machine Learning models.
In Part 4, I’ll focus on the Machine Learning model.
Thank you for reading! Follow me here and on social media to make sure you don’t miss the next installment. If you found this article useful, a share and some claps would mean the world to me and help fuel the rest of my series.
Questions or comments? I’d be more than happy to answer them here or via email.
You can also find me on LinkedIn, Facebook, Instagram, and my personal website.